Symbolic AI and Ontology for Regulatory Affairs
Published on May 19, 2025 — 4 min read
Last week, a LinkedIn post caught my eye: a regulatory team leaned on ChatGPT to assess whether an OTC drug met U.S. compliance requirements and reached an incorrect conclusion due to outdated information. In regulatory affairs—the unsung guardian of company reputation, public safety, and market trust—errors like this can have dire consequences. The incident is a reminder that while large language models, or any probabilistic models in general, can boost productivity and speed up processes, their use must be cautious when precision is non-negotiable.
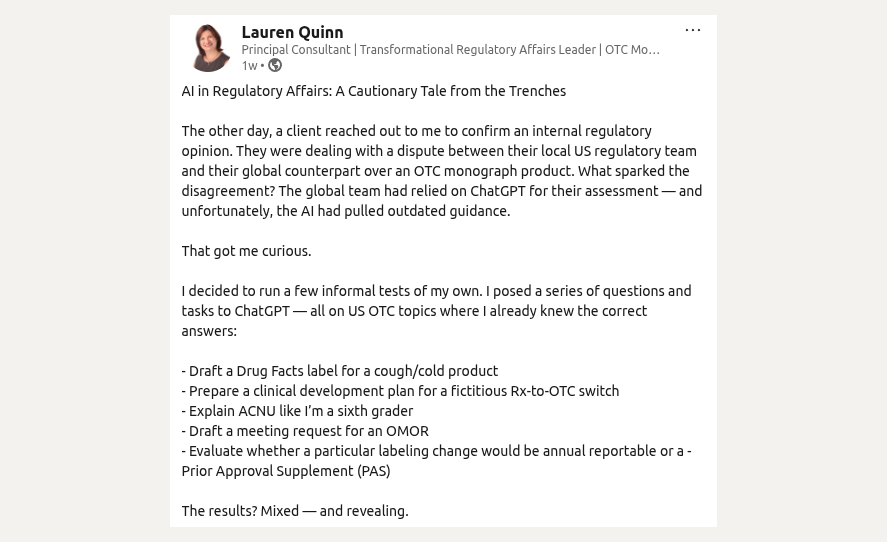
Is AI a poor fit for regulatory affairs? Not necessarily. In fact, there is another branch of AI that may be better suited for regulatory affairs: Symbolic AI. Instead of finding patterns in data, it works with explicitly defined rules and concepts, and uses logical reasoning, much like a mathematical proof, to reach conclusions in a deterministic and explainable way:
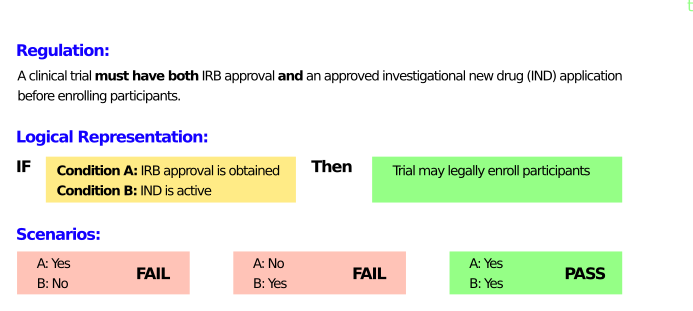
One of the most powerful tools in the symbolic AI toolbox is ontology—structured, machine-readable representations of a given domain, including its concepts, relationships, and rules. In regulatory contexts, an ontology provides a systematic way to model legal requirements, business processes, roles, and obligations in a way that reflects their real-world meaning. But beyond just organizing information, modern ontologies also come with mechanisms—such as rule engines, consistency checking, and reasoning capabilities—that allow systems to perform automated assessments.
Here’s how these ideas are being explored in academic and early-stage pilot projects:
Pharmaceutical
In pharma, ontology has been used to translate complex regulatory expectations into precise, machine-checkable rules. The SPuMoNI project, for example, modeled the ALCOA data integrity principles as a formal ontology and used it to automatically flag issues, such as timestamp inconsistencies in real-time manufacturing data. Similarly, ontology-based SHACL and SKOS have been combined to validate chemical safety data sheets against international hazard phrase lists, enabling automated rechecks whenever standards are updated.
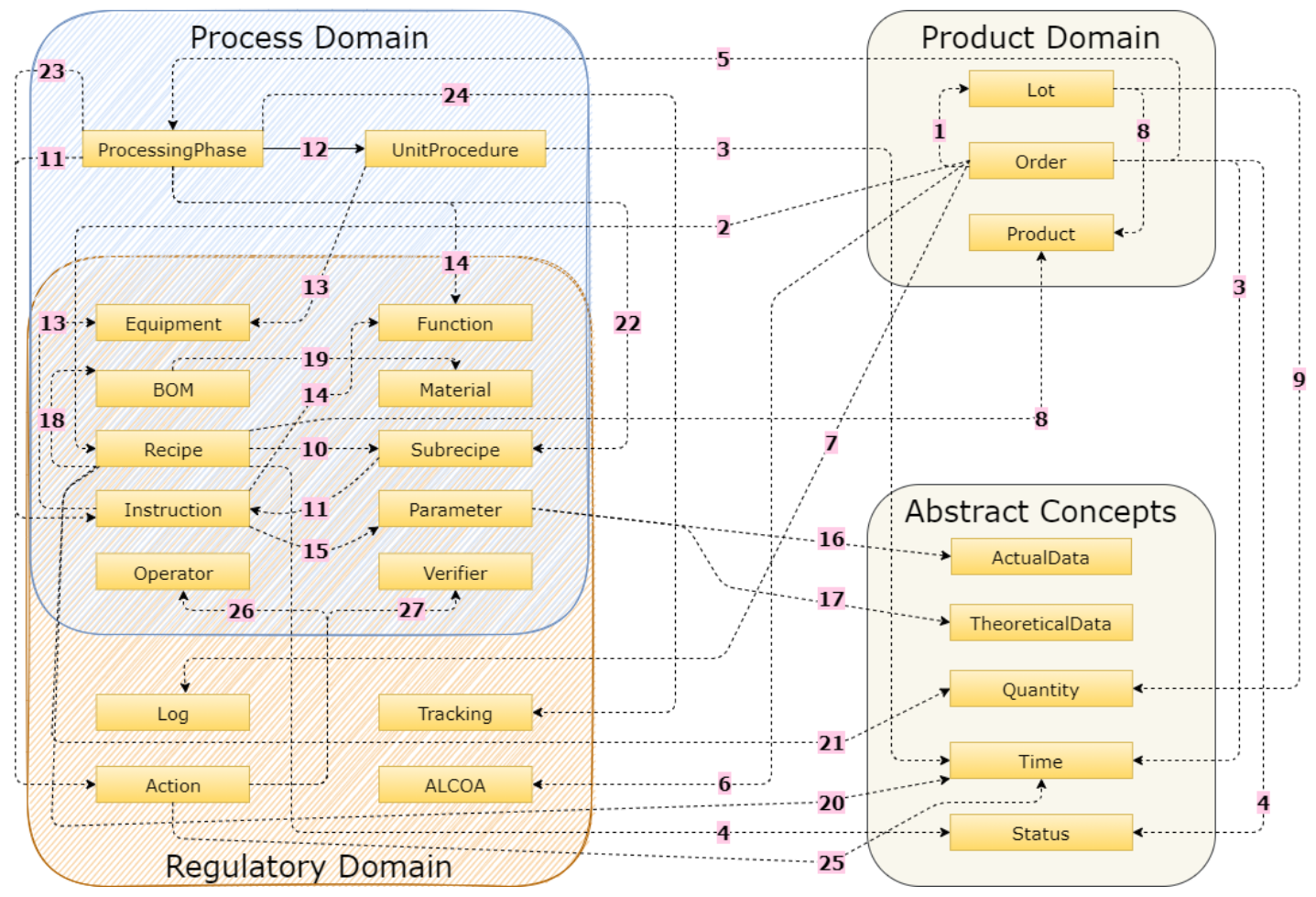
Construction
In the construction sector, researchers have applied ontologies to encode building codes into databases for automated review of architectural plans. One study focused on compliance check system for fire safety and accessibility violations in buildings. Another project converted ISO 23386 standard into an ontology and used it for continuous, structured compliance checks in building information modeling.
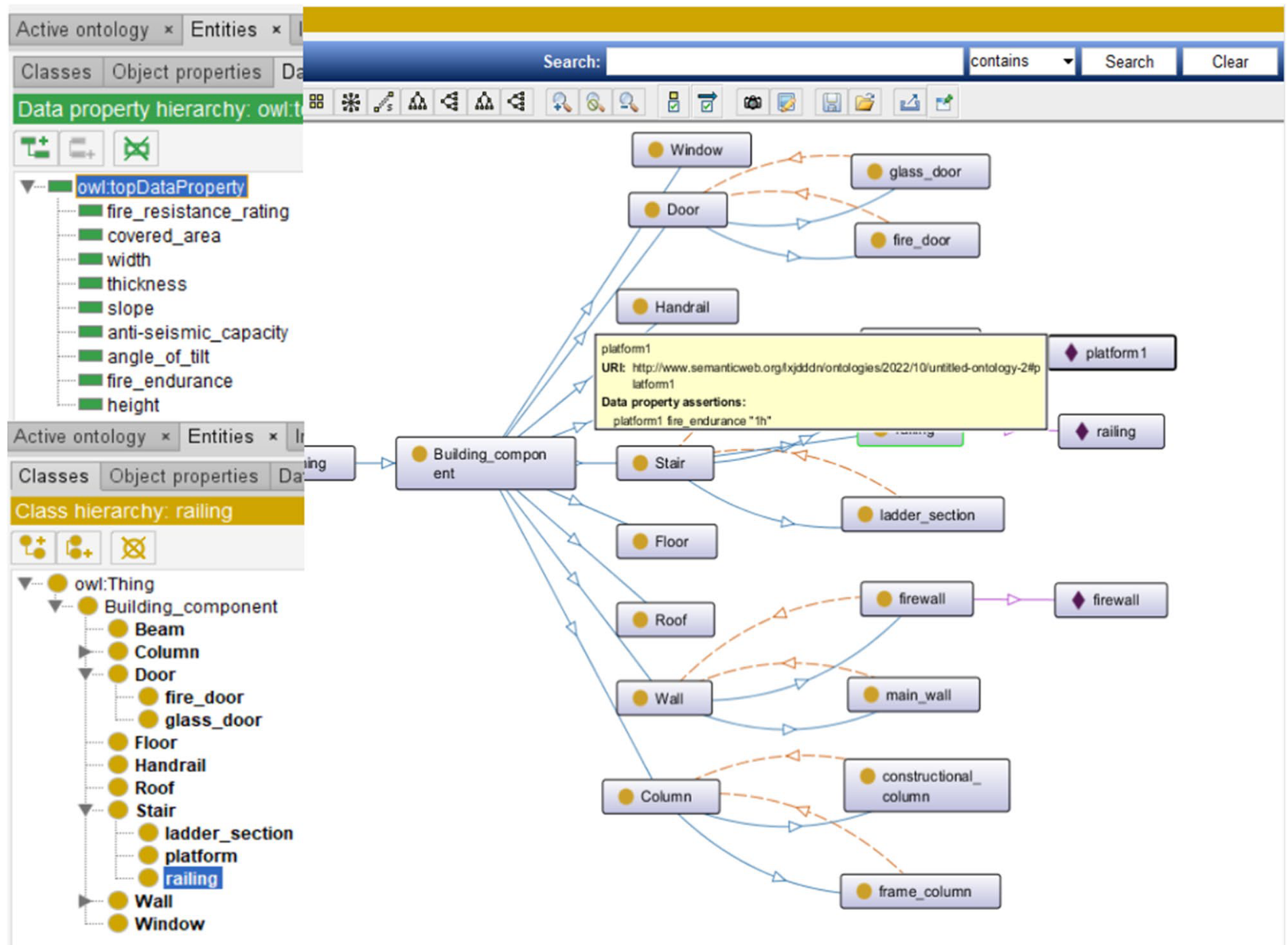
Transportation
In transportation, the Norwegian Maritime Authority has modeled regulatory requirements as SHACL constraints to automatically determine certificate eligibility for vessels and personnel. In the automotive space, safety engineering teams have used ontologies and logic rules to perform risk assessment under ISO 26262 for vehicle functional safety.
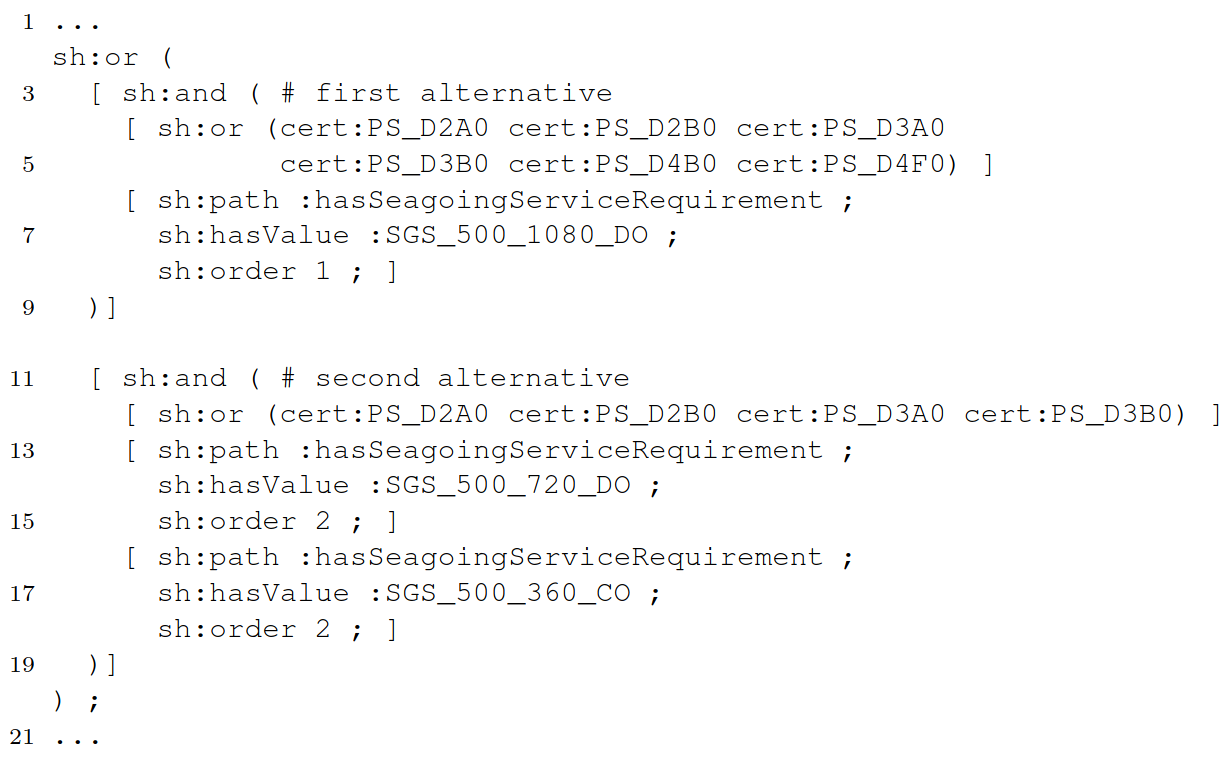
To summarize, for regulatory affairs professionals, I invite you to explore the lesser-known but promising direction of symbolic AI and ontology. Unlike ChatGPT, this approach is grounded in logic and structure well suited for encoding regulatory rules in a way that’s both auditable and explainable. If your organization is starting to explore AI for regulatory workflows, it may be worth asking: could there be a role for this approach too?
- OntologySymbolic AIData ManagementRegulatory Assessments