Ontology-Based Data Management for Industrial R&D — Insights from Novo Nordisk
Published on April 14, 2025 — 3 min read
Industrial R&D scientists often work with large amounts of heterogeneous data across multiple isolated systems, so-called "data silos". This fragmented setup can lead to inconsistencies and difficulties in data integration. How can organizations develop a data strategy that handles diverse sources and evolving technologies, while still ensures data quality and fosters effective collaboration across teams and departments?
One proposed solution is to use an ontology—a structured map of domain knowledge that defines key concepts and how they relate to each other, in a format both humans and computers can understand. Extensive public ontologies already exist—particularly in biomedical research—that provide standardized concepts and relations as building blocks. By adopting this shared language, different systems and teams can better align their data, streamline integration and promote reuse.
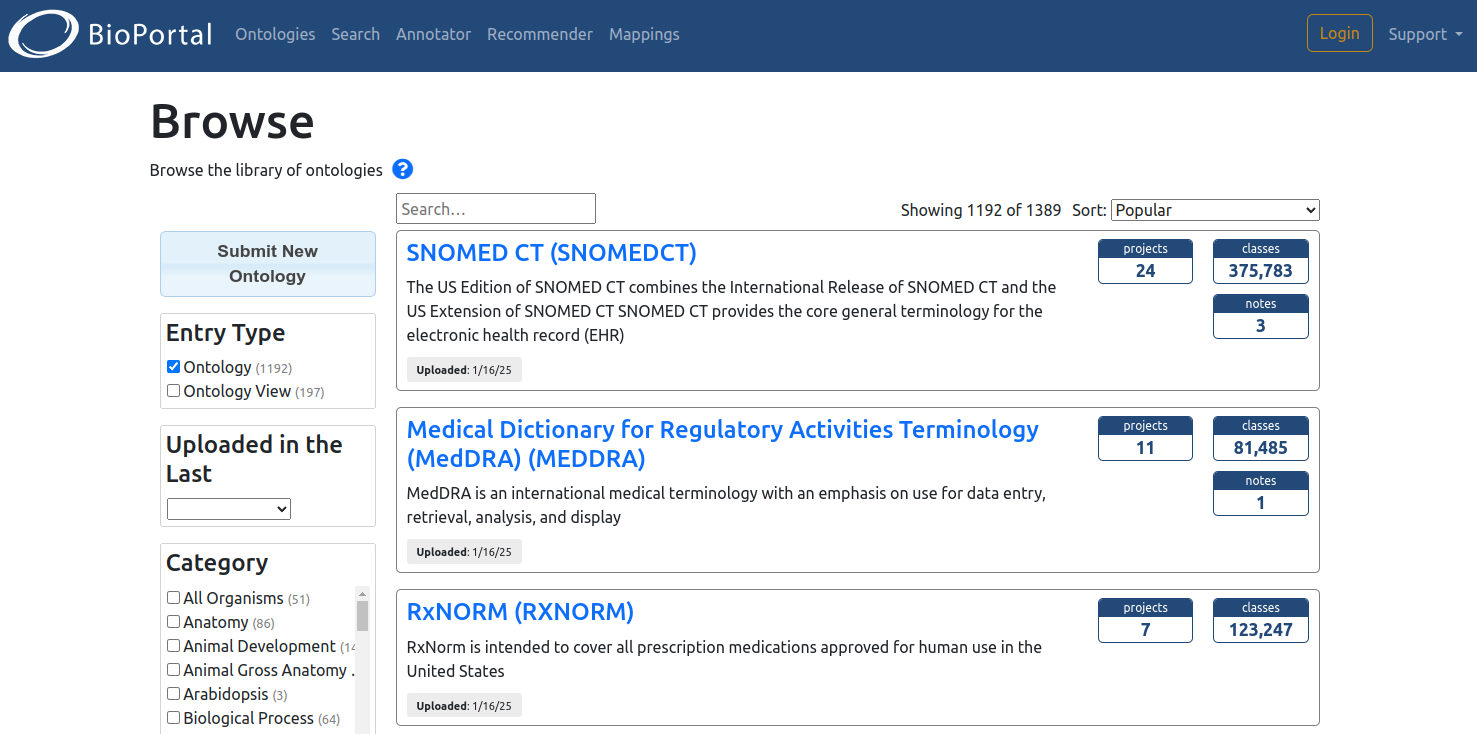
In a recent publication in the Journal of Biomedical Semantics (Digital Evolution: Novo Nordisk’s Shift to Ontology-Based Data Management, Tan et al., 2025), Novo Nordisk shared how they address R&D data challenges with their ontology-based data management system (OBDM). The system features a unified R&D data model, a set of controlled vocabularies for internal software, and a knowledge graph that provides a comprehensive view of all relevant data.
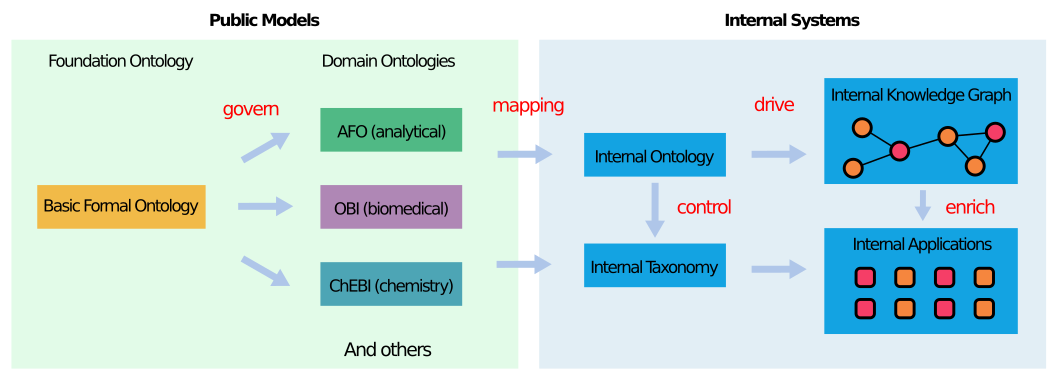
ODBM's data model is based on established public ontologies (including Allotrope Foundation Ontology, Ontology for Biomedical Investigations, Chemical Entities of Biological Interest). Although these public resources offer broad, community-driven standards, they do not always match a company’s internal terminology or processes. To bridge this gap, the authors developed automated methods to merge, harmonize and map public ontologies into Novo Nordisk's model. The outcome is a cohesive, enterprise-ready knowledge framework that preserves alignment with external standards while fulfilling the immediate data needs of the organization’s R&D efforts.
To guide consistent data input, the ontology is used to create controlled vocabularies that are integrated into Novo Nordisk's internal R&D software—for example, populating dropdown menus. This approach simplifies how data is entered, improves its quality, and helps ensure that different teams use the same language when working with information. Usage metrics show that increasing numbers of teams across the organization are adopting these standardized vocabularies, suggesting clear benefits in data consistency and integration.
Another key element of Novo Nordisk’s system is the virtual knowledge graph (VKG). While knowledge graphs enhance data discovery and collaboration, building and maintaining them can be time-consuming. By using a VKG, Novo Nordisk provides a graph-based query interface on top of existing data sources, all driven by the unified ontological data model. This method ensures data stays current without extensive duplication, which is critical for compliance, governance, and timely decision-making in pharmaceutical R&D.
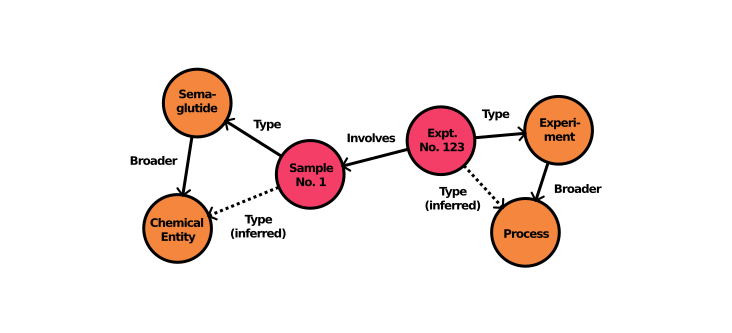
In summary, Novo Nordisk’s work highlights both the feasibility and strategic value of ontology-based data management in large, data-intensive R&D environments. Their journey provides valuable insights into ontology orchestration, taxonomy dvevelopment, knowledge graphs, and practical AI integrations. By sharing practical methods for overcoming real-world challenges, Novo Nordisk offers lessons that any organization seeking a robust, scalable, and future-proof data strategy can readily adopt.
- Data StrategyOntologyKnowledge GraphSPARQL